New Computer Learning Tool 95% Accurate in Screening for SSc
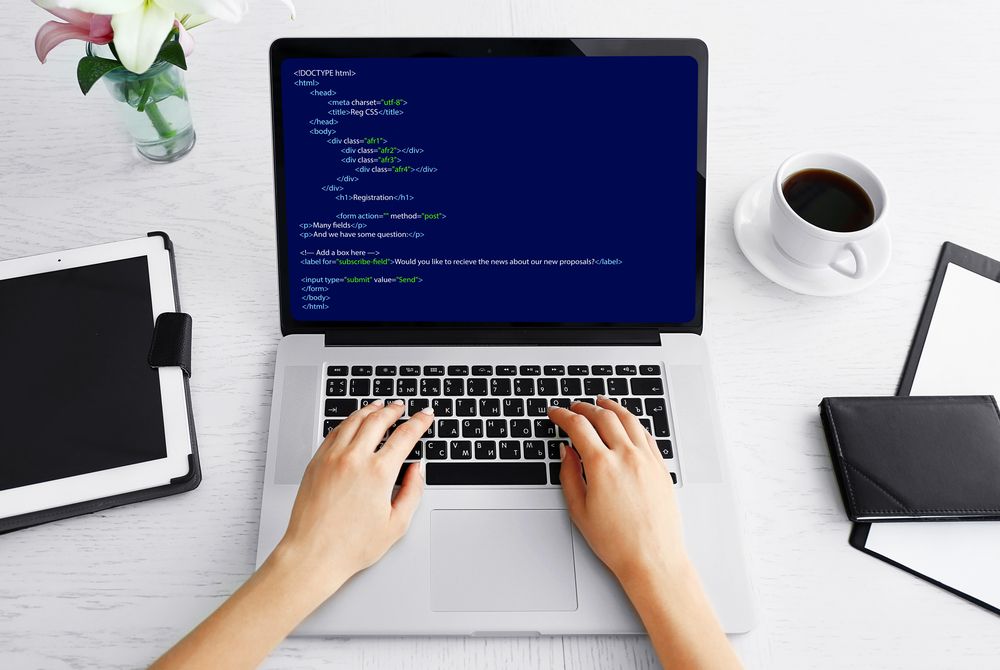
Using a laptop, a computer-based machine learning tool was able to distinguish, with 95% accuracy, normal skin images from those collected from people with systemic sclerosis (SSc), a study demonstrated.
This tool has the potential to rapidly diagnose SSc and to accurately classify the extent of the condition, the scientists said.
Moreover, it could “easily be implemented in a clinical setting, providing a simple, inexpensive, and accurate screening tool,” according to the researchers.
The study, “Deep Learning Classification of Systemic Sclerosis Skin using the MobileNetV2 Model,” was published in the journal Engineering in Medicine and Biology.
SSc, also called systemic scleroderma, is an autoimmune disease characterized by widespread scar tissue accumulation in the skin and internal organs. Because there are a variety of SSc symptoms, early diagnosis and determining the extent of disease progression can pose significant challenges for clinicians and may result in treatment and disease management delays.
Thus, there is a need for objective and consistent methods to diagnose SSc.
Computer-based machine learning techniques already are widely used to process and analyze large amounts of data generated for various purposes. Machine learning has shown potential in diagnosing autoimmune diseases, in predicting outcomes, and in classifying disorders such as rheumatoid arthritis, inflammatory bowel disease, and lupus.
Now, scientists from the biomedical engineering department at the University of Houston have used a type of machine learning called deep neural networks to analyze and learn from skin images collected from SSc patients and healthy individuals to predict the presence and extent of SSc.
“Our preliminary study, intended to show the efficacy of the proposed network architecture, holds promise in the characterization of SSc,” Metin Akay, PhD, founding chair of the biomedical engineering department and the study’s lead author, said in a university press release.
“By scanning the images, the network learns from the existing images and decides which new image is normal or in an early or late stage of disease,” Akay said.
Deep learning organizes computer programs known as algorithms into layers that can learn and make decisions. The team used a deep learning network called convolutional neural networks (CNNs), commonly used in engineering, medicine, and biology.
By combining a modified CNN architecture (UNet) with extra algorithm layers, the team developed a new mobile training module called MobileNetV2.
Notably, the proposed network was used on a standard laptop computer.
The training phase of the network included 834 images from 20 people without scleroderma and 678 images from 20 early and late-stage SSc patients, for a total of 1,512 images. A validation phase used 104 normal and 84 SSc images.
“By scanning the images, the network learns from the existing images and decides which new image is normal or in an early or late stage of disease,” Akay said.
Taking less than five hours to complete, the training phase diagnosed all of the 1,512 image sets with no misdiagnosis, yielding an accuracy of 100% and a sensitivity of 100%. A test’s sensitivity is its ability to correctly identify a disease.
In the validation phase, the networks correctly diagnosed 81 of 84 SSc image sets and misdiagnosed three, yielding a sensitivity of 96.4%. Of note, 101 of the 104 normal images were correctly classified, with an overall accuracy of 96.8% and a specificity of 97.1%. Of note, specificity is a test’s ability to correctly identify people without a disease.
The final testing phase — analyzing 104 normal and 84 SSc images — correctly diagnosed 80 of 84 SSc image sets with a sensitivity of 95.2%. It also correctly diagnosed 99 of the 104 normal images, yielding an overall accuracy of 95.2% and specificity of 95.1%.
As a comparison, the team ran the unmodified CNN architecture on the same laptop. The process took more than 14 hours to train and validated 65 of 84 SSc images with a sensitivity of 77.3%. Likewise, the testing phase correctly diagnosed 60 of 84 SSc images, yielding a sensitivity of 71.4%.
“These results indicated that the MobileNetV2 architecture is more accurate and efficient compared to the CNN to classify normal and SSc skin images,” the team wrote.
To distinguish between early and late-stage SSc, an additional 1,887 images — 1,041 normal, 423 early stage SSc, and 423 late-stage SSc — were analyzed. The validation phase correctly diagnosed 178 of 183 normal, early, and late-stage SSc images, yielding an overall accuracy of 97.2%.
Two of the 40 early stage images were misclassified as late-stage, while another two were misclassified as normal. Additionally, one of the 103 normal images was misclassified as early stage SSc. All 40 late-stage SSc were correctly diagnosed.
Consistently, the testing phase correctly diagnosed 182 of 192 normal, early, and late-stage SSc images, yielding an overall accuracy of 94.8%. Again, the unmodified CNN architecture was less accurate in identifying different SSc stages.
“Our ultimate goal is to use this approach as a rapid and reliable method to assess the severity of SSc using images,” the researchers wrote. “The proposed network architecture could assign a high-accuracy diagnosis within minutes. This saves substantial time and money, compared to how diagnoses are currently made.”
“We believe that the proposed network architecture could easily be implemented in a clinical setting,” Akay concluded, noting that it is “a simple, inexpensive, and accurate screening tool for SSc.”