Genetic Profile Models Show Potential to Predict Person’s Scleroderma Risk
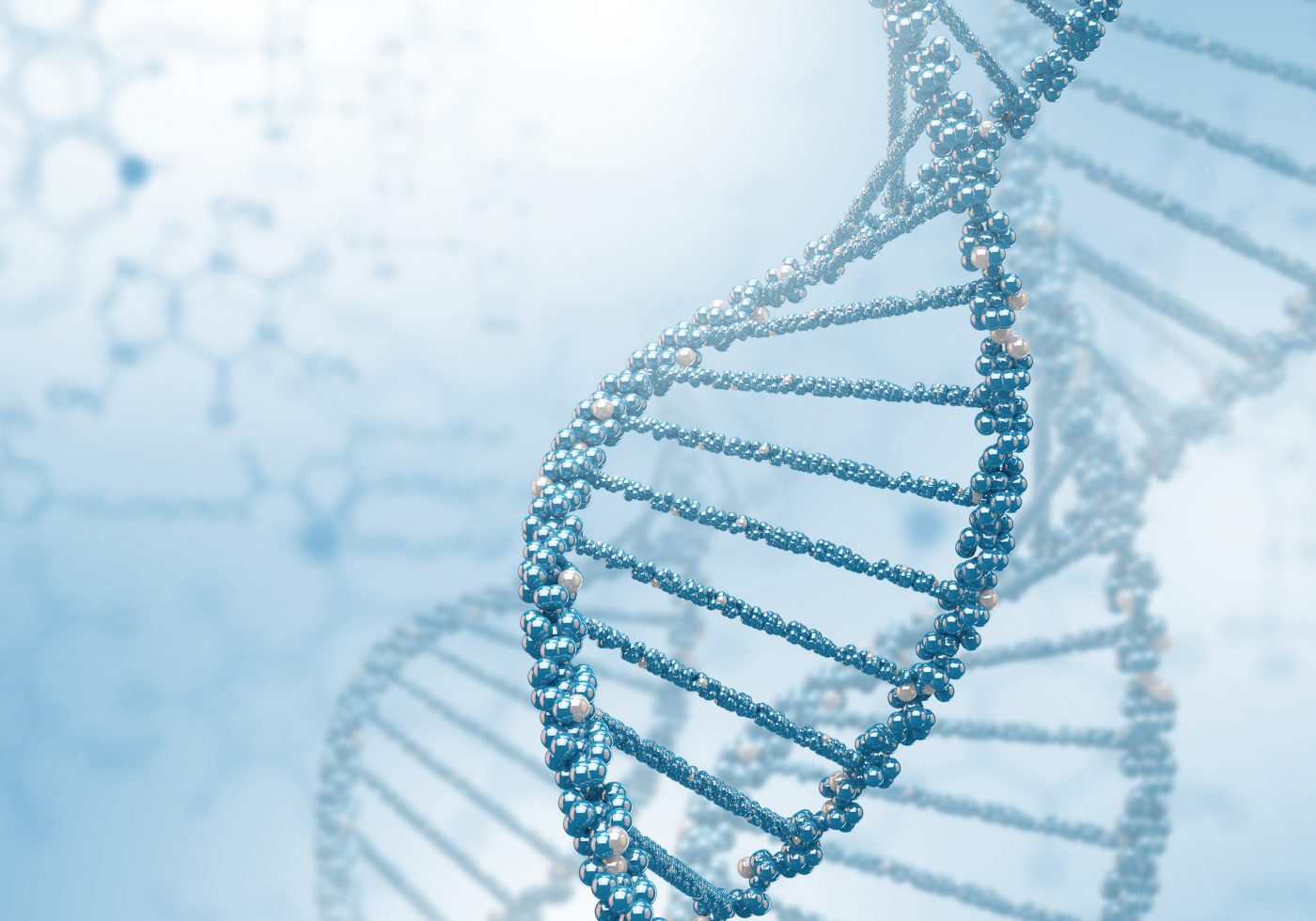
Genomic risk scores (GRS), which predict the likelihood of developing a condition based on a person’s genetic profile, successfully distinguished people with scleroderma from those with other immune-mediated inflammatory diseases or healthy individuals, a study reported.
The investigation, which marks the first use of a GRS model to predict scleroderma risk, found that including age and immune cell counts in the model significantly increased its prediction accuracy. But more work is needed, its researchers advised.
The study, “Genomic Risk Score impact on susceptibility to systemic sclerosis,” was published in the journal Annals of the Rheumatic Diseases.
Scleroderma, an inflammatory disease affecting the connective tissue, is characterized by hardened skin and complications in the heart, lungs, kidney, and digestive system. It belongs to a group of immune-mediated inflammatory disorders, arising from genetic and environmental factors. These apparently unrelated disorders share many clinical characteristics and, with the advent of genome-wide association studies, common genetic factors have also been identified.
Data from such studies can be used to predict the risk of developing a given disease by analyzing an individual’s genetic profile, looking for variations associated with that disease. Genomic risk scores (GRS) use these data to combine the effects of all disease-related genetic factors, weighted by strength of association, into a disease risk prediction.
GRS has been used successfully to predict risk for neuropsychiatric and cardiovascular conditions, as well as some immune-mediated inflammatory disorders. However, it has not previously been used to predict scleroderma risk.
An international research team sought to develop a GRS model to accurately predict this risk, and identify patients at high risk, allowing for earlier diagnosis and treatment of a disease whose symptoms vary widely depending on its severity.
Scleroderma can be classified based on extent of fibrosis (hardening or scarring of tissue) and by the presence of scleroderma-specific autoantibodies, or antibodies that attack a patient’s own cells. Anti-centromere antibodies (ACA) are generally associated with limited cutaneous scleroderma, and anti-topoisomerase antibodies (ATA) with diffuse systemic scleroderma.
Notably, topoisomerase-I is a key enzyme for the winding of DNA molecules during gene expression and cell division; the centromere is a part of chromosomes.
Using data collected in large GWAS, the risk model was developed with data from the 518 non-Spanish patients in the European PRECISESADS study, and tested in the 339 Spanish patients. GRS was calculated in an independent group of 400 scleroderma patients and 571 healthy people of European ancestry serving as controls.
The best-fitting model captured the effects of 33 disease-associated single nucleotide polymorphisms (SNPs) — genetic variations in which a single building block of DNA is changed — and had a 67% chance of accurately distinguishing between scleroderma patients and controls. The GRS was also able to differentiate between patients with three other immune-mediated inflammatory diseases — rheumatoid arthritis, Sjögren’s syndrome, and systemic lupus erythematosus — and controls, although with less accuracy than scleroderma.
Significantly, the GRS distinguished scleroderma patients from those with rheumatoid arthritis in 56.8% of the cases, and from people with Sjögren’s syndrome in 58.5% of the cases. Lupus and scleroderma have a common genetic component, which made it impossible to accurately differentiate between these disorders.
The model did not distinguish between localized and systemic scleroderma, so the researchers used genomic data to compare people by clinical and autoantibody subtype. The clinical GRS classified patients into limited or diffuse cutaneous scleroderma with modest accuracy, while the autoantibody subtype GRS distinguished between ATA- and ACA-positive patients with a higher degree of accuracy.
Immune cell counts also contributed to the prediction model. When combined with age, four immune cell subtypes — including activated dendritic cells and memory B-cells — independently improved risk prediction accuracy. A model that includes age, GRS, and cell count was more accurate than without such parameters, suggesting that clinical and demographic information are also helpful.
Altogether, “we consider that the reported GRS could enhance SSc [scleroderma] diagnosis in the future and may contribute to personalised medicine, as a tool to assist physicians in the diagnosis of SSc,” the researchers wrote.
According to the investigators, the study’s limitations included the relatively small size of the groups involved.
“The sensitivity and specificity of the model are still far from clinical use and it will require the addition of extra information and/or the development of well-powered phenotype [manifestation]-specific GWAS,” the team added.